- Authors*
Emmanuel Doumard, Julien Aligon, Elodie Escriva, Jean-Baptiste Excoffier, Paul Monsarrat, Chantal Soulé-Dupuy
*Détails des auteurs accessibles sur la publication
- Reference
Edinburgh, UK - March 29, 2022
The most widely used additive explanation methods, SHAP and LIME, for understanding the predictions of complex machine learning models suffer from limitations that are rarely measured in the literature. This paper aims at measuring these limitations. We illustrate and validate the results on a specific medical dataset, SA-Heart.
Our results reveal that LIME and SHAP approximations are particularly efficient in high dimension and generate intelligible global explanations, but they suffer from a lack of precision regarding local explanations. The coalition-based methods are computationally expensive in high dimension, but provide better local explanations. Finally, we present a roadmap summarizing our work by indicating the most appropriate method depending on the dimension of the dataset and the user's goals.
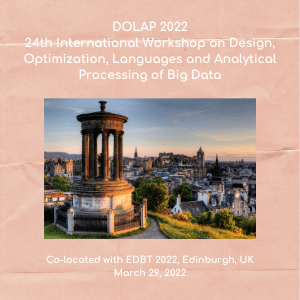