Noémie Salaün-Penquer - Data scientist Kaduceo
In France, according to ATIH reports (French for Technical Agency of Information about Hospitalization), 12% of patients hospitalised in 2017 were readmitted within 30-day. Predicting hospital readmissions might help health professionals to identify high-risk patient clusters and so put measures in place to improve healthcare quality.
Medico-administrative data, from the PMSI (French for Program of Information System Medicalization) contains various information about patients (gender, age, place of residence), the stay (healthcare facility, length of stay, mode of entry and exit, diagnoses, procedures made during the stay) and information about economic valorization (homogeneous group of patients, surcharge). These data constitute an important database to investigate factors that might influence these readmissions. These data have already been used to predict readmission risk in a French context (7,11, ...)
Predicting readmissions is of a real interest and is addressed by several actors in global way.
To day and as far as we know, there is no unique and reliable model to identify patient at high risk of readmission.
Hospital Readmission: healthcare quality indicator
Studying hospital readmission might contribute to improve healthcare pathways but the subject is quite complex. The different models in the scientific literature are difficult to compare. To predict the readmission risk, a specific part of the population is often targeted. For example, the pathologies for which the highest readmission rate has been observed are: heart failure, pneumonia, liver disease and acute myocardial infarction. Elderly persons have also a higher readmission risk (6, 13, 14, ...). Researchers focus, most of the time, one of these populations or several at the same time or some other populations of particular interest at a given time. Data used to develop models are mainly medico-administrative data, but these are often incomplete or poorly filled and difficult to compare across hospitals, regions, countries. Furthermore, some limitations might avoid to reach the optimal quality of the model. It has been demonstrated that the distinction between planned and unplanned readmissions affects the results of these models (12, 17), but it is difficult to make this distinction within the PMSI data. It is also difficult to prove a clear link between the patient’s first stay and their readmission (7, 11).
Taking into consideration these limitations, machine learning could contribute to extract as much information as possible from available data. Machine learning could provide important insights about patient at high-risk readmission and thus help hospitals and health professionals to better deal with them.
The subject of hospital readmission has been of interest to healthcare professionals since the early 1980s. These readmissions soon began to be considered as healthcare quality indicator in the United States and elsewhere (1, 2, 3, 5). Models most commonly cited in the scientific literature to predict readmission risk are logistic regression models as well as proportional hazards models including Cox models. The latter take into account the time before an event occurs (such as the readmission) as well as patients not readmitted in the period studied (for example in a 30-day period) as censored data. These types of models have the advantage to be easily explainable because they allow inferences to be made not only on the readmission risk but also the effect of the different factors analyzed (6, …, 13, 17, 42). The first attempts for readmission risk modelling, until around 2011, gave very modest results regarding discrimination quality of the models developed (c-statistic below 0.70 for most of the models). However, more recent modelling attempts have resulted in a c-statistic between 0.75 and 0.92 for some populations (17, 42).
0 %30-day readmission
Relevant factors:
Patient medical history
Length of stay
Comorbidities
Age
Studies on this subject have allow to highlight some relevant factors that would influence hospital readmissions. Among these; the most cited are: patient’s medical history (for example several hospitalisations in the year before the stay studied), the length of stay as well as patient comorbidities. Based on these studies, indicators of readmission risk have been developed. In particular, the PARR index, developed in United Kingdom, includes 7 factors exploring age, previous hospitalisations, comorbidities and precarity, but its sensitivity is very low to detect 30-day readmissions (22, 17, 43). The LACE index, developed in Canada, includes 4 factors exploring length of hospitalisation, accident and emergency admission, number of comorbidities, number of accident and emergency visits in the last 6 months. It has been approved to detect the risk of 30-day and 90-day readmissions for heart failure patients and for general population but still has difficulties to make accurate predictions for all the conditions and populations (34, 35, 36, 37, 38, 17, 43). These indicators use estimates of the coefficients related to each factor through logistic regression to compute a risk score for each patient.
In addition to logistic models, others machine learning methods have been used or improved in order to predict patient’s risk of early readmission. To mention some of them, Support Vector Machines (SVM) or decision trees which use a hierarchical representation of data structure in the form of decision sequences (test) in order to predict a numerical value or a class (9, 18, 20, 21, 42). These models are not yet widely used but could provide interesting results.
Artificial Intelligence to go further
Artificial intelligence might further the research on the prediction of hospitalisation readmissions. Deep learning models, are more and more used for this purpose and usually obtain better results than other types of models (23, .., 30). Their structures are complex but research on explainability of the results provided is actively beginning. These researches on explainability are inspired by image recognition, a field that already makes the most of deep learning. Methods for evaluating the importance of the factor are being developed: factors are compared to pixels of a picture. The model is built iteratively, removing factors one by one and thus the importance of the information that each factor provides is estimated (31, 32).
Recent comparative studies show that results obtained with the different models still have a too wide variability (c-statistic which can vary from 0.21 to 0.92 according to studies). Most of the models used are classical statistical models (logistic regression). In the future, it is expected to see more and more machine learning models to deal with this topic. Some of the most recent models have achieved to be very discriminant (17, 40, 41, 42). However these models sometimes lack validation (internal and/or external). It is therefore difficult to generalise to other populations than those studied. The two models that reach a c-statistic above 0.80 (40, 41) try to differentiate planned and unplanned readmissions and focus on readmissions that are considered as planned.
However, this distinction is very difficult to make (17, 42, 44, 45), especially with PMSI data. Another reason for the improvement in the quality of the models over the years may be linked to the fact that the 30-day period has been considered as most relevant for hospital readmission. Indeed, previous studies included readmission period that varied between 7 days and 2 years and thus often neglected development of the patients over the time. Nevertheless results remain globally rather modest (17, 39, 42). The latest comparative reviews that take into account studies published after 2011 highlight only 10 models out of 73 with a c-statistic above 0.70 (17) and around 19% of models with a c-statistic above 0.75 for a more recent review (42).
Readmission risk modeling
At KADUCEO, we are interested in readmission risk modelling. We are working on several methods that we apply to data from PMSI while integrating external data in order to enrich our models as much as possible. For example, we have integrated meteorological data (temperature, wind, visibilty, etc.) with the medico-administrative data. We believe that new machine learning methods could be applied to address this problem, in association with classical models, in order to obtain a good quality of prediction while keeping a good explainability.
Our journey design begins with the first recording of a patient for a reason in a healthcare facility until the
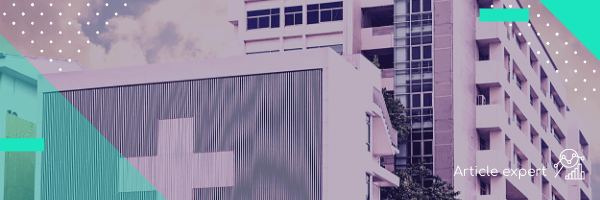
State of the art: Prediction of hospital readmission
The study of hospital readmission could contribute to the improvement of care paths but the subject is quite complex. The
Bibliography
1. Jencks SF, Williams M, Coleman MV.Rehospitalizations among patients in the medicare fee-for service program. N EnglJ Med 2009;360:10.2 – Centers for Medicare and Medicaid Services.
2. Community-based care transitions program. http://innovation.cms.gov /initiatives / CCTP /, visité 2019.
3. Blanc, Anne-Laure & Fumeaux, Thierry & Stirneman, Jérôme & Bonnabry, Pascal & Schaad, Nicolas. (2017). Hospital readmissions : Current problems & perspectives. Revue Medicale Suisse. 13. 117-120.
4. Bahrami, S., Holstein, J., Chatellier, G., Le, Y. R., & Dormont, B. (2008). Using administrative data to assess the impact of length of stay on readmissions: study of two procedures in surgery and obstetrics. Revue d’epidemiologie et de sante publique, 56(2), 79-85.
5. OR, Z., & RENAUD, T. (2009). Quel lien entre volume d’activité des hôpitaux et qualité des soins en France?. Questions d’économie de la santé, (149), 1-6.
6. Kansagara D, Englander H, Salanitro A, et al. Risk prediction models for hospital readmission: a systematic review. JAMA. 2011;306(15):1688-98.
7. Yilmaz, E., & Vuagnat, A. (2015). Tarification à l’activité et réadmission. Économie et Statistique, 475(1), 71-87.
8. Fisher SR, Graham JE, Krishnan S, Ottenbacher KJ. Predictors of 30-Day Readmission Following Inpatient Rehabilitation for Patients at High Risk for Hospital Readmission. Phys Ther. 2015;96(1):62-70.
9. Frizzell JD, Liang L, Schulte PJ, et al. Prediction of 30-Day All-Cause Readmissions in Patients Hospitalized for Heart Failure: Comparison of Machine Learning and Other Statistical Approaches. JAMA Cardiol. 2017;2(2):204–209. doi:10.1001/jamacardio.2016.3956
10. Zhao P, Yoo I (2017) A Systematic Review of Highly Generalizable Risk Factors for Unplanned 30-Day All-Cause Hospital Readmissions. J Health Med Informat 8:283. doi: 10.4172/2157-7420.1000283
11. Mercier, G., Spence, J., Ferreira, C., Delay, J. M., Meunier, C., Millat, B., … & Seguret, F. (2018). Postoperative Rehabilitation May Reduce the Risk of Readmission After Groin Hernia Repair. Scientific reports, 8.
12. Hansen LO et al. Interventions to reduce 30-day rehospitalization: a systematic re-view. Ann Intern Med 2011;155(8):520-8.
13 .Franchi C et al. Risk factors for hospital readmission of elderly patients. Eur J In-tern Med 2013;24(1):45-51. 54.
14. Dharmarajan K et al. Diagnoses and Timing of 30-Day Readmissions After Hospitalization for Heart Failure, Acute Myocardial Infarction, or Pneumonia.JAMA 2013;309(4):355-363.
15 . Robinson, R., & Hudali, T. (2017). The HOSPITAL score and LACE index as predictors of 30 day readmission in a retrospective study at a university-affiliated community hospital. PeerJ, 5, e3137.
16 .Garrison GM, Robelia PM, Pecina JL, Dawson NL. Comparing performance of 30-day readmission risk classifiers among hospitalized primary care patients. Journal of Evaluation in Clinical Practice. 2016 doi: 10.1111/jep.12656.
17. Zhou H, Della PR, Roberts P, Goh L, Dhaliwal SS. Utility of models to predict 28-day or 30-day unplanned hospital readmissions: an updated systematic review. BMJ Open. 2016;6(6):e011060. doi: 10.1136/bmjopen-2016-011060
18. Cui, S., Wang, D., Wang, Y., Yu, P. W., & Jin, Y. (2018). An improved support vector machine-based diabetic readmission prediction. Computer methods and programs in biomedicine, 166, 123-135.
19. Fuhrman, C., Moutengou, E., Roche, N., & Delmas, M. C. (2017). Prognostic factors after hospitalization for COPD exacerbation. Revue des maladies respiratoires, 34(1), 1-18.
20 . Lee, E. W. (2012). Selecting the best prediction model for readmission. Journal of Preventive Medicine and Public Health, 45(4), 259.
21 . Natale, J., & Wang, S. (2013). A decision tree model for predicting heart failure patient readmissions. In IIE Annual Conference. Proceedings (p. 3518). Institute of Industrial and Systems Engineers (IISE).
22.Billings, J., Blunt, I., Steventon, A., Georghiou, T., Lewis, G., & Bardsley, M. (2012). Development of a predictive model to identify inpatients at risk of re-admission within 30 days of discharge (PARR-30). BMJ open, 2(4), e001667.
23. Berry, J. G., Gay, J. C., Maddox, K. J., Coleman, E. A., Bucholz, E. M., O’Neill, M. R., … & Hall, M. (2018). Age trends in 30 day hospital readmissions: US national retrospective analysis. bmj, 360, k497.
24. Maali Y, Perez-Concha O, Coiera E, Roffe D, Day RO, Gallego B. Predicting 7-day, 30-day and 60-day all-cause unplanned readmission: a case study of a Sydney hospital. BMC Med Inform Decis Mak. 2018;18(1):1. Published 2018 Jan 4. doi:10.1186/s12911-017-0580-8
25. Donzé J, Aujesky D, Williams D, Schnipper JL. Potentially avoidable 30-day hospital readmissions in medical patients: derivation and validation of a prediction model. JAMA Intern Med. 2013;173(8):632–638. doi: 10.1001/jamainternmed.2013.3023.
26. Donzé JD, Williams MV, Robinson EJ, Zimlichman E, Aujesky D, Vasilevskis EE, Kripalani S, Metlay JP, Wallington T, Fletcher GS, Auerbach AD, Schnipper JL. International validity of the HOSPITAL score to predict 30-day potentially avoidable hospital readmissions. JAMA Internal Medicine. 2016;176(4):496–502. doi: 10.1001/jamainternmed.2015.8462.
27. Nguyen, P., Tran, T., Wickramasinghe, N., & Venkatesh, S. (2017). $mathtt {Deppr} $: A Convolutional Net for Medical Records. IEEE journal of biomedical and health informatics, 21(1), 22-30. (https://arxiv.org/pdf/1607.07519.pdf)
28. Rajkomar, A., Oren, E., Chen, K., Dai, A. M., Hajaj, N., Hardt, M., … & Sundberg, P. (2018). Scalable and accurate deep learning with electronic health records. npj Digital Medicine, 1(1), 18.
29. Golas, S. B., Shibahara, T., Agboola, S., Otaki, H., Sato, J., Nakae, T., … & Kvedar, J. (2018). A machine learning model to predict the risk of 30-day readmissions in patients with heart failure: a retrospective analysis of electronic medical records data. BMC medical informatics and decision making, 18(1), 44.
30. Wang, H., Cui, Z., Chen, Y., Avidan, M., Abdallah, A. B., & Kronzer, A. (2018). Predicting hospital readmission via cost-sensitive deep learning. IEEE/ACM Transactions on Computational Biology and Bioinformatics (TCBB), 15(6), 1968-1978.
31. Xiao, C., Ma, T., Dieng, A. B., Blei, D. M., & Wang, F. (2018). Readmission prediction via deep contextual embedding of clinical concepts. PloS one, 13(4), e0195024.
32. https://www.ncbi.nlm.nih.gov/pmc/articles/PMC5890980/
32. Suresh, H., Hunt, N., Johnson, A., Celi, L. A., Szolovits, P., & Ghassemi, M. (2017). Clinical Intervention Prediction and Understanding using Deep Networks. arXiv preprint arXiv:1705.08498.
33. Avati, A., Jung, K., Harman, S., Downing, L., Ng, A., & Shah, N. H. (2018). Improving palliative care with deep learning. BMC medical informatics and decision making, 18(4), 122.
34 . Cotter, P. E., Bhalla, V. K., Wallis, S. J., & Biram, R. W. (2012). Predicting readmissions: poor performance of the LACE index in an older UK population. Age and ageing, 41(6), 784-789.
35 . Robinson, R., & Hudali, T. (2017). The HOSPITAL score and LACE index as predictors of 30 day readmission in a retrospective study at a university-affiliated community hospital. PeerJ, 5, e3137.
36 . van Walraven, C., Wong, J., & Forster, A. J. (2012). LACE+ index: extension of a validated index to predict early death or urgent readmission after hospital discharge using administrative data. Open Medicine, 6(3), e80.
37 . van Walraven, C., Dhalla, I. A., Bell, C., Etchells, E., Stiell, I. G., Zarnke, K., … & Forster, A. J. (2010). Derivation and validation of an index to predict early death or unplanned readmission after discharge from hospital to the community. Cmaj, 182(6), 551-557.
38 . Aubert, C. E., Folly, A., Mancinetti, M., Hayoz, D., & Donzé, J. (2016). Prospective validation and adaptation of the HOSPITAL score to predict high risk of unplanned readmission of medical patients. Swiss medical weekly, 146, w14335.
39 . Ross, J. S., Mulvey, G. K., Stauffer, B., Patlolla, V., Bernheim, S. M., Keenan, P. S., & Krumholz, H. M. (2008). Statistical models and patient predictors of readmission for heart failure: a systematic review. Archives of internal medicine, 168(13), 1371-1386.
40. Donzé, J., Lipsitz, S., & Schnipper, J. L. (2014). Risk factors for potentially avoidable readmissions due to end‐of‐life care issues. Journal of hospital medicine, 9(5), 310-314.
41. Shams, I., Ajorlou, S., & Yang, K. (2015). A predictive analytics approach to reducing 30-day avoidable readmissions among patients with heart failure, acute myocardial infarction, pneumonia, or COPD. Health care management science, 18(1), 19-34.
42. Artetxe, A., Beristain, A., & Grana, M. (2018). Predictive models for hospital readmission risk: A systematic review of methods. Computer methods and programs in biomedicine.
43. Baig, M., Zhang, E., Robinson, R., Ullah, E., & Whitakker, R. (2018). Evaluation of Patients at Risk of Hospital Readmission (PARR) and LACE Risk Score for New Zealand Context. Studies in health technology and informatics, 252, 21-26.
44. Van Walraven, C., Bennett, C., Jennings, A., Austin, P. C., & Forster, A. J. (2011). Proportion of hospital readmissions deemed avoidable: a systematic review. Cmaj, 183(7), E391-E402.
45. Benoit Besse. Réadmissions à 30 jours par le service des urgences : fréquence, pertinence et déterminants de la prise en charge à l’aide de deux grilles d’évaluation. Médecine humaine et pathologie.2014